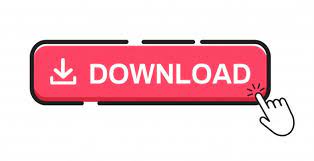
Second, electronic inefficiencies during reading and converting the electrical charge into a digital count exhibit another type of noise, namely read noise. Which is the result of random variation of the detected photons. Firstly, the sensor readings are corrupted with shot noise Ĭonstructed a convolutional autoencoder which was able to jointly design CFA and demosaick, therefore they obtained a CFA that out-performed the common Bayer CFA for image reconstruction purposes.Īpart from the demosaicking problem, another problem that requires special attention is the elimination of noise arising from the sensor and which distorts the acquired raw data. an ensemble of CNNs was developed which contained different models trained to demosaick patches with specific attributes, for example, textures and smooth areas, while Henz et al. Consequently, new CNN approaches were developed extending the usage of CNNs in the field. Įxploit the advantages in the field of deep learning to create a deep Convolutional Neural Network (CNN) that is able to demosaick images, and a lot of effort was put by the authors to create a new large demosaicking dataset, namely the MIT Demosaicking Dataset which consists of 2.6 million patches of images. In earlier works feed forward neural networks were used on par with dictionary methods in order to obtain adaptive solutions for image demosaicking, while in small patches were used to train a multi-layer neural network minimizing an error function. The effectiveness of neural networks for image demosaicking has been studied for over a decade. Furthermore, two popular CFA patterns are contained into the dataset, namely the Bayer and Fuji X Trans, which permits the development and evaluation of methods that can deal with different CFA patterns. The reason is that the contained images have to be demosaicked in the linear RGB (linRGB) color space of the camera before being transformed via color transformation and gamma correction into standard RGB (sRGB) space that common consumer display devices use. We use the produced Microsoft Demosaicking dataset to train, evaluate and compare our system. In their work, they thoroughly explained a methodology to create a demosaick dataset which is on par with reality. proposed a way to produce a dataset with realistic reference images allowing for the design of machine learning demosaicking algorithms. Under this training strategy, the main issue is that demosaicking artifacts on the training data will hinder the performance and the overall quality of the reconstruction. they apply a mosaick mask on the already demosaicked images, therefore obtaining non-realistic pairs for tuning trainable methods. In many approaches such as those in the authors used already processed images as references that are simulated mosaicked again, i.e.
#Denoiser iii not enough ram full#
READ FULL TEXT VIEW PDFĭuring recent years, research is directed towards learning based approaches, although a common problem with the design of learning based demosaicking algorithms is the lack of ground-truth images. Trained by using a significantly smaller number of training data than existingĭeep demosaicking networks. Than the current state-of-the-art solution and furthermore can be efficiently Network architecture, which as a result requires fewer trainable parameters In reconstruction quality is attributed to the principled way we design our Our extensive experimentation lineĭemonstrates that our proposed network outperforms any previous approaches onīoth noisy and noise-free data across many different datasets. Consequently, ourĭerived neural network has a transparent and clear interpretation compared to Large-scale optimization and deep learning techniques. Recently introduced to solve this problem, in this work we propose a novelĪlgorithm which is inspired by powerful classical image regularization methods, While there are several machine learning systems that have been Two-thirds of the intensity information are missing and the rest are perturbedīy noise. Modern approaches try to jointly solve these problems, i.e jointĭenoising-demosaicking which is an inherently ill-posed problem given that
#Denoiser iii not enough ram series#
Related to denoising and demosaicking where the former aims to reduce noiseįrom the sensor and the latter converts a series of light intensity readings toĬolor images.
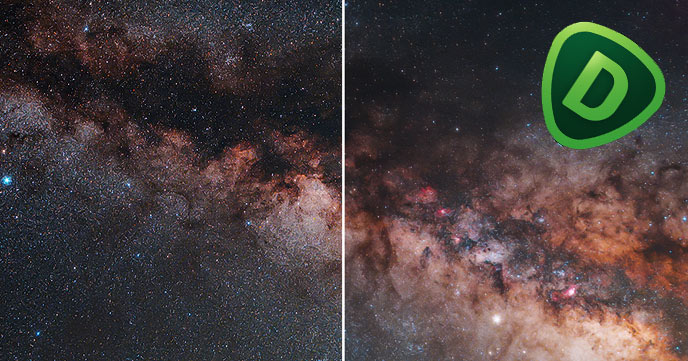
Processing steps to produce realistic images. Modern digital cameras rely on sequential execution of separate image
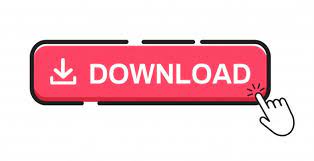